In recent years, artificial intelligence has captivated the public’s attention and sparked widespread curiosity.
Generative AI in the form of ChatGPT, DALL-E, and other interactive technologies have made headlines for their ability to generate human-like responses, create art, write stories, and even simulate thousands of adoring fans.
While these innovations have brought the potential of AI to the forefront of everyday conversations, the technology behind them has been around for decades.
“A lot of the technologies that we use every day on our phones and computers were considered artificial intelligence at one point,” explained Neil Chilson. “The fact that you can interact with ChatGPT is what makes people think of it differently than, say, an app on their phone, but the underlying technology is actually quite similar.”
Chilson, former chief technologist at the Federal Trade Commission, currently heads AI policy at the Abundance Institute, a nonprofit focused on removing barriers to emerging technologies.
While AI’s rapid growth presents tremendous opportunities, it also raises important questions. According to Chilson, the key to unlocking AI’s true potential is experimentation, much like the early days of electricity when trial and error revealed the full impact of the shift.
Only by using technology in real-world scenarios can we begin to understand its capacity to address complex challenges.
Here, Chilson provides a comprehensive look at the many facets of generative AI, offering a grounded perspective on its current and future impact.
This conversation has been edited for length and clarity.
Artificial intelligence is surprisingly hard to define. Can you give it a shot?
Artificial intelligence as a technology has been around forever. It has gone through lots of different versions. The best way to think about AI is that it’s the most cutting-edge computer science of the time.
When I was a kid getting interested in computers, the cutting-edge, PhD-level research in artificial intelligence was teaching computers to play chess, and now we all have apps on our phones that play chess better than 99.9% of all humans. A lot of the technologies we use every day on our phones were called artificial intelligence at some point. Google’s search engine, text recognition, and facial recognition were all considered cutting-edge AI not too long ago.
John McCarthy, who coined the term “artificial intelligence” in the 1950s used to say, “As soon as it works, no one calls it AI anymore.” They just call it technology.
What is generative AI? How is it new or different from the AI we’ve been using already?
Generative AI is unique because it creates content based on a user prompt. The main technology behind ChatGPT or DALL-E is a large language model (LLM) that has learned to generate human-like language by analyzing vast amounts of data. When a user asks it a question, the technology predicts which words would logically follow to make sense in that context. What surprises many, even the developers, is how sophisticated the generated content can be. The responses seem intuitive.
The actual technology for LLMs has existed since the 1970s, but it turns out you need massive amounts of data for them to be useful. The internet provided that because we’ve all been posting pictures of, say, our avocado toast online. You need a lot of pictures of avocado toast as well as a lot of accompanying text for an LLM to understand what avocado toast is — that it’s avocado on a piece of toast instead of something else.
Compared to humans, generative AI requires huge amounts of data to learn. Humans need far fewer examples, handle more complex sensory information, and understand context better than computers.
Sign up for the Stand Together newsletter and get stories, ideas, and advice from changemakers to help you tackle America’s biggest problems.
What potential do you see in this technology?
Generative AI is a general-purpose technology, much like computers, which are now used in everything from microwaves to analyzing star clusters. This technology represents a new, though not brand-new, form of computation. Traditional programming involves humans providing instructions. LLMs learn from massive datasets, effectively programming themselves by recognizing patterns.
When I think about the future of AI and how this is going to impact people’s lives, I look to the spaces where we can collect tons of data, but we don’t necessarily know what the data means yet. We’re not really sure how it connects to the real world.
In health care, for example, AI is being used to detect cancer months earlier than trained doctors. While a skilled radiologist might examine 10,000 or 100,000 scans during their training, an LLM can analyze millions of scans, drawing on that vast data each time it reviews a new one. In the process, it identifies patterns and makes connections within the data that humans simply can’t see.
Logistics is another great example. Let’s say you have a very complicated network of providers moving products. Machine learning could analyze your logistics and suggest more efficient routes and resource allocation.
What impact do you see generative AI having on the economy?
I like to think of AI as a tool that magnifies intellectual labor.
The previous wave of automation was about physical jobs, factory jobs. When robots automated routines and repetitive tasks, those jobs shifted. The jobs in factories became more about overseeing or maintaining robots. I think we’ll see something similar in how these tools will automate big chunks of intellectual labor, things like data analytics, content generation, and software engineering.
The result will be increased productivity in certain roles.
For a software engineer, rather than spending all day debugging a piece of tricky code, they can feed it into an AI that has seen tons of bugs, and it can point the engineer in the right direction quickly. Without those time-consuming menial tasks, software programming will become easier, and we’ll be able to direct that energy toward solving more difficult problems or problems that used to be too expensive to fix.
Anytime there’s an increase in productivity, jobs change. We can expect that in certain sectors people will change their jobs.
Saying, ‘If a job is easier, you’ll need fewer people to do it (and fewer jobs),’ is an oversimplification. While fewer people may be needed for the same job, making a task easier can have a broader impact on the economy. It opens up opportunities for more people to enter that field, adapt to the changes, and discover new ways to leverage the technology.
There seem to be many unknowns about generative AI and its potential impact, which might feel risky to some. Can you provide a framework for handling these uncertainties?
We sometimes get stuck in this mindset of, ‘We have to figure out all the potential applications and risks before we let people use this.’ The truth is, especially with general-purpose technologies like AI, you’ll never know until people start applying it to the problems they’re trying to solve.
When electricity first came out, factories at the time were run on a single steam engine in the center of a large production floor. The engine had belts and pulleys that went all over the place, powering the different tools around the room. They all ran on this one machine.
When factory owners adopted electricity, they just replaced the steam engine with a single electric motor that still ran all the machines in the room. They didn’t really change the structure, just the power source.
But then what? People started to realize they could make small electric motors and put them in individual machines. Then they weren’t dependent on this one big motor that could halt all productivity in the factory. They didn’t need the belts and chains running all over the place. Eventually, they decentralized everything. Electricity totally restructured how factories worked, what the jobs were like, and how dangerous they were.
No one could have predicted that. It only happened because people tried it. That’s how it’s going to play out in this space, too. The uncertainty is not just in where the current technological path is, but also how it gets deployed. It’s really hard to imagine until people sit down and try to use it themselves, when they say, “What problem am I facing, and how will this help me?”
The number of problems we can solve with these powerful general-purpose technologies is, well, we don’t really know how boundless it is.
How can we leverage generative AI to have a greater social impact?
The most obvious answer here goes back to the idea that generative AI is a tool that magnifies our intellectual labor. These tools make it possible for small nonprofits to achieve more with fewer resources. AI tools can help streamline tasks like content creation, strategy development, and outreach, which would typically require hiring consultants or experts. This allows them to expand their impact and reach more people, even with limited budgets or staff.
There are likely many other areas in the social impact space where this technology’s ability to scale and reach more people could have a significant impact. Just like with anything else, the key is going to be experimentation. We’ll discover generative AI’s social benefits as we create them.
Abundance Institute is supported by Stand Together Trust, which provides funding and strategic capabilities to innovators, scholars, and social entrepreneurs to develop new and better ways to tackle America’s biggest problems.
Learn more about Stand Together’s efforts to make the economy work for all and explore ways you can partner with us.
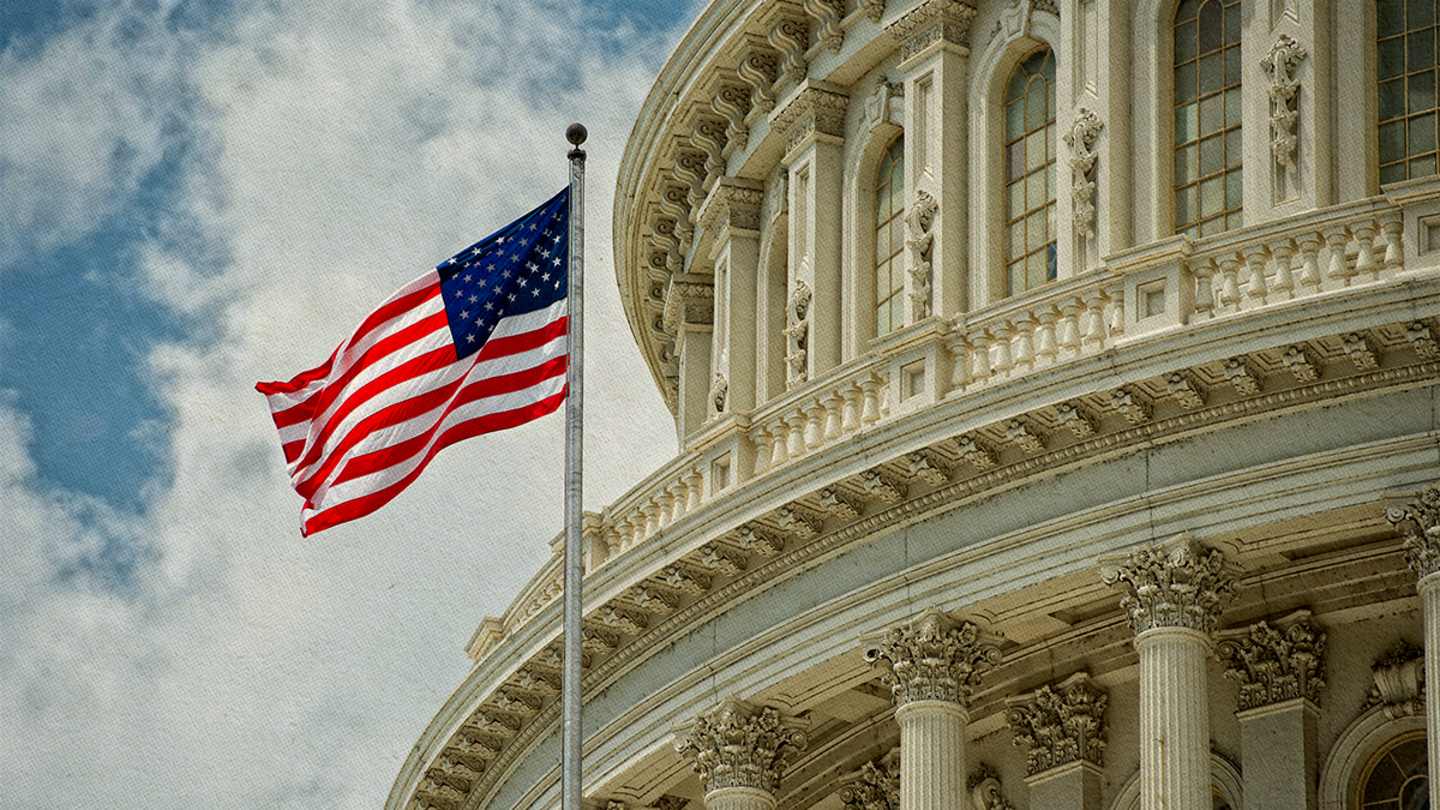
Here are some issues the Department of Government Efficiency could target.
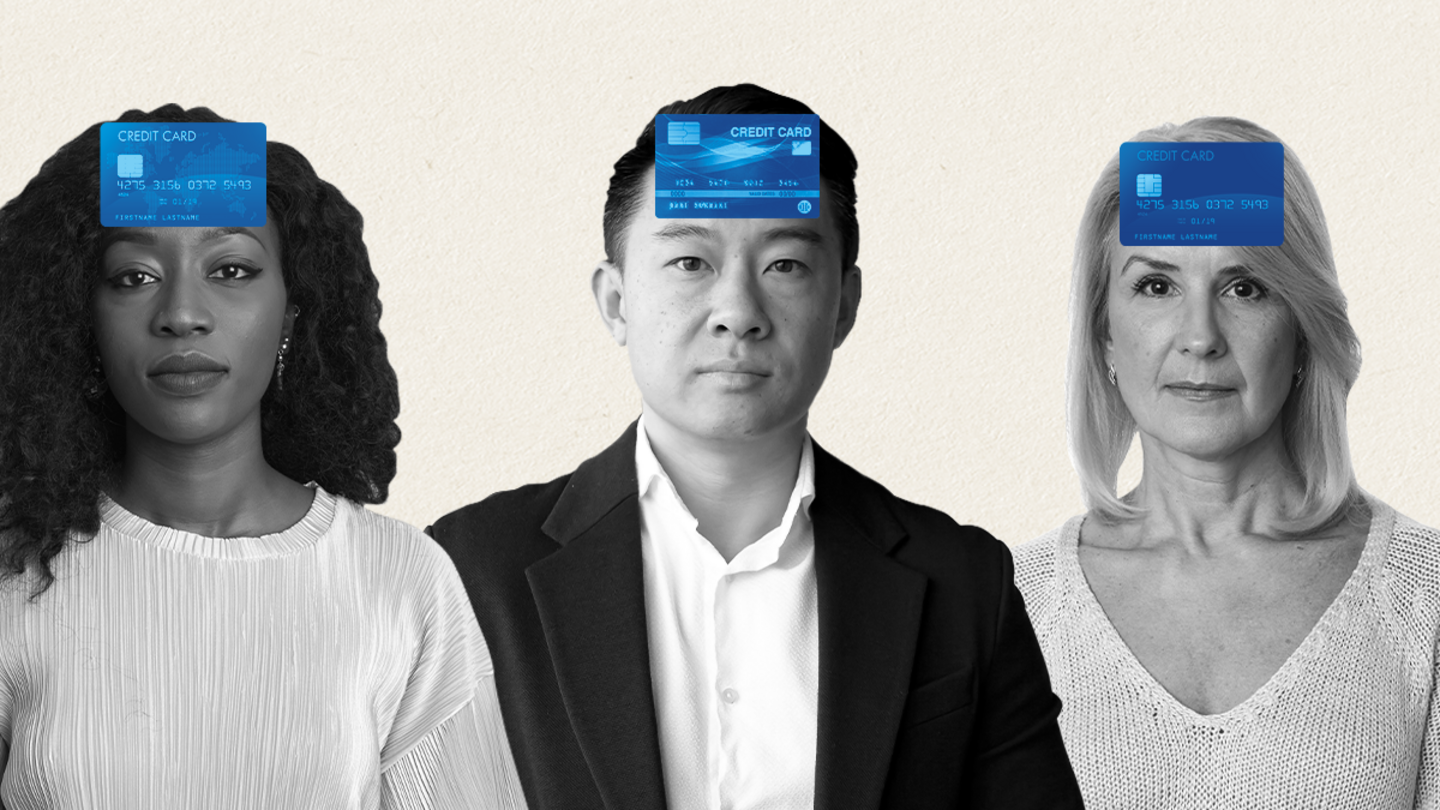
Credit scores don’t reflect the whole person. Here’s one way to change the narrative.
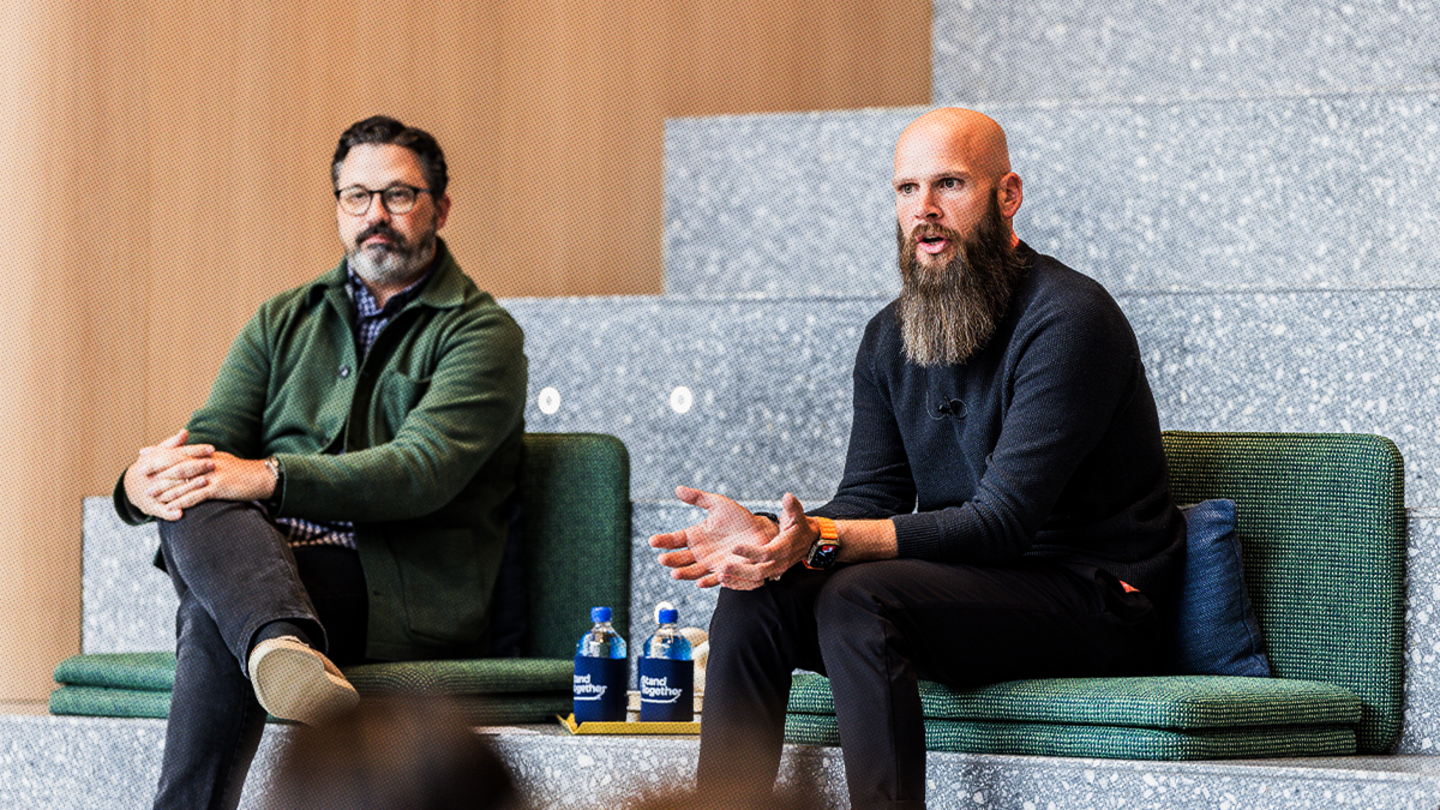
Fear of change is nothing new, but how we respond to this moment really matters.
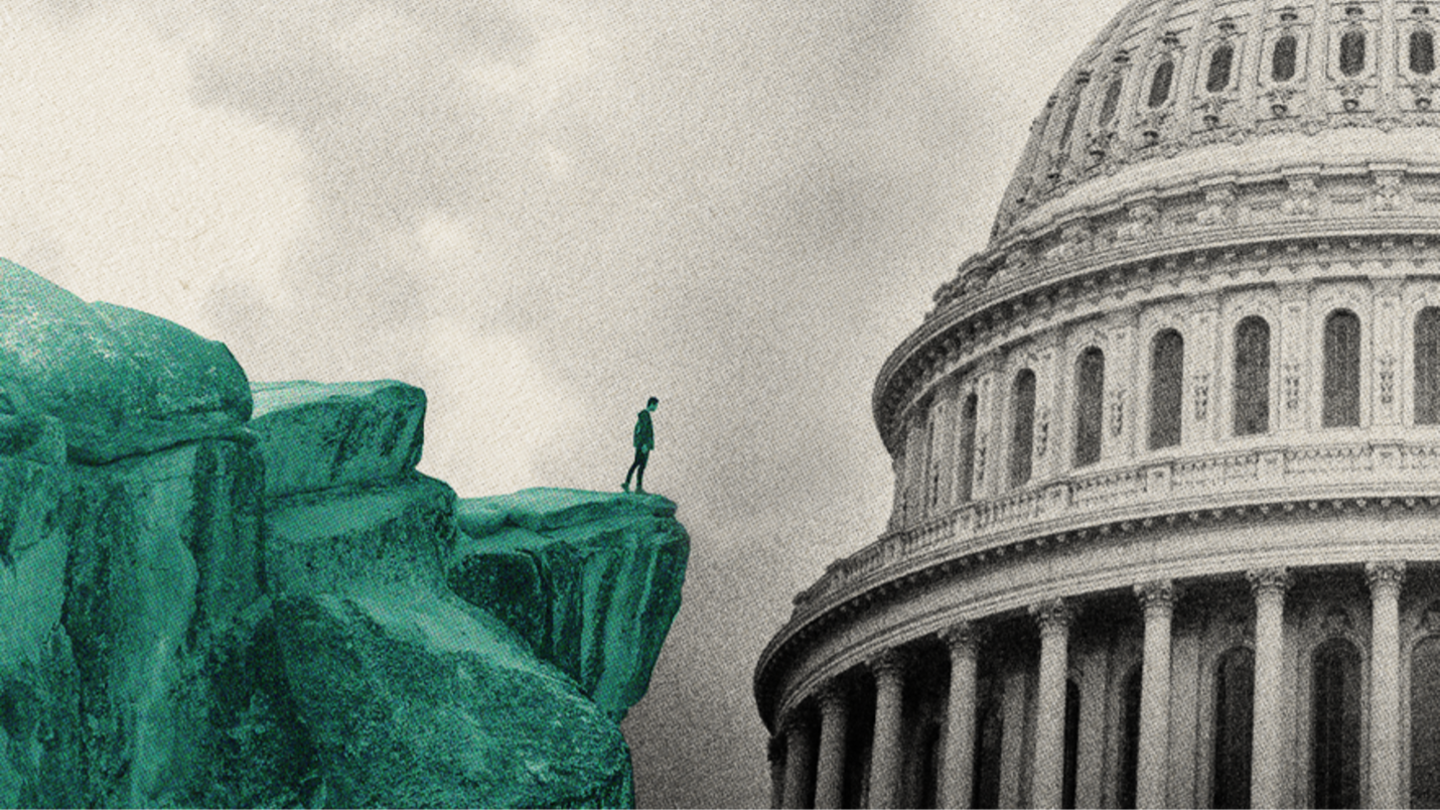
A coalition of scholars and policymakers are working on long-term economic solutions — together.